What is AI Personalization?
Artificial intelligence personalization (AI personalization) is a technology that uses machine learning algorithms to adapt or tailor information or experience specifically to suit an individual’s needs, preferences, and characteristics. AI personalization has become an increasingly important trend in Web, m-commerce, and interactive gaming.
The method looks at the user, their interests and preferences, and their past interactions with the brand. Based on these data points, a machine learning algorithm can predict what information or experiences will make users engage more fully with the platform over time.
How businesses are using AI personalization?
AI personalization is popular in different fields such as e-commerce, online gaming, travel, hospitality, and more, where the goal is to design an experience and product offerings that will appeal to end users, who are increasingly mobile and engaged with multiple programs and devices.
The main benefits of AI personalization are improved user experiences, higher conversion rates, and enhanced user trust. AI personalization can also help organizations gather better customer information to provide targeted experiences through personalized content and insights into user preferences and behaviors through data mining.
In addition, AI personalization can also help save costs for organizations by reducing the number of staff needed in marketing and customer service departments.
E-commerce and gaming organizations use AI personalization to analyze past transactions and interactions with their users and user profiles to provide content or recommendations similar in type or style to an individual’s previous selections.
Travel companies use AI personalization to provide their customers personalized travel experiences by tailoring content and recommendations to suit their needs.
Examples of AI personalization in eCommerce
One common example of AI personalization is the Amazon recommendation system, which recommends books, music, clothing, and other items. This system uses information about the customer’s purchase history and a machine-learning algorithm to predict products likely to interest the customer.
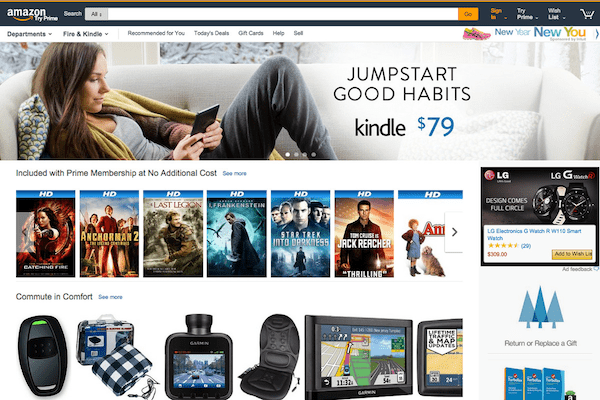
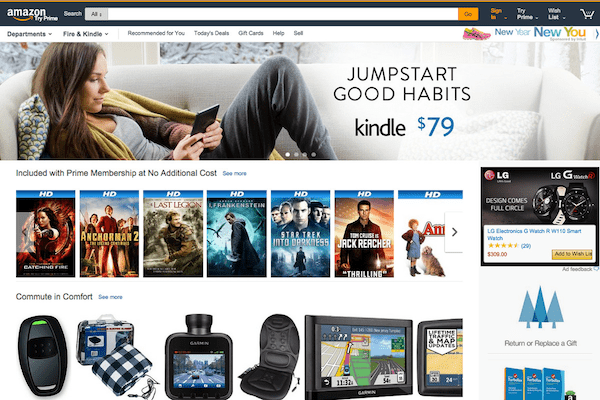
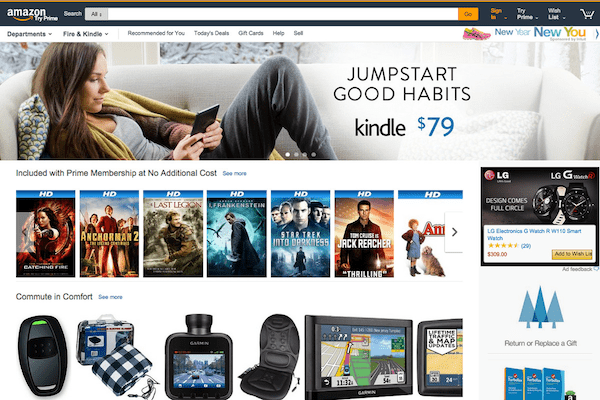
Recommendations are not only based on the actual content of purchase history but also on other factors such as who else bought it, how often they bought it, and how they rated the product. For example, if a customer buys a book about dog training, Amazon might suggest other books on hounds. And if a customer has rated another book highly, Amazon may recommend that book to another reader.
Another well-known use of AI personalization is Netflix. The company’s recommendation engine turns a person’s viewing habits into a personalized mix of movies and TV shows.
This system analyzes what customers have watched and what they rate as often watched. For example, suppose the customer has enjoyed an action movie. In that case, they will likely enjoy another action movie. In contrast, customers who liked romantic comedies might be more likely to enjoy romantic comedies again.
How to optimize your eCommerce website with AI personalization
1. Strategy and objectives
It is important to define the purpose and objectives of the AI personalization project. As with any technology, there are many possible uses for it. Key questions to consider include: Who are we trying to reach? Why are we doing this? How do we want users to experience our website, platform, or application? What will result from this experience? What will it entail in terms of cost, human resources, and revenue generation?
2. Data and analysis
AI personalization can be effective in reaching a large number of users. However, before rolling out a system, it is important to consider the rights and ownership of the information that your site will collect.
For example, your website must declare the use of user data for creating profiles (e.g., collecting loyalty card data).
The legal concerns may include preventing discriminatory advertising against specific groups of people or limiting access to certain users based on their data, such as age or geographic location.
In addition to privacy and data protection, users may be concerned about the accuracy of data captured and ultimately used in an algorithm. This concern can be due to more transparency over data collection and analysis. A better solution is allowing users to control which data they want to share with the algorithm.
3. Core capabilities
Next, it is important to define the corporate AI system’s capabilities.
Identification: This is identifying individuals based on text or other information. The factors that determine the identification include the type of data analyzed in the profile, the amount of data available, and whether your website uses machine learning or other techniques to analyze the data.
Image recognition: This is commonly known as facial recognition. It can identify a person from their face in an image based on key facial features such as eyes, mouth, and body structure (e.g., hair and clothes).
Search and recommendation: This provides the user with specific results tailored to a query or a profile. These can include targeted adverts and suggestions of similar items or products that may interest the user.
Natural language processing: This refers to the ability of a computer to understand human language and respond in kind. NLP is useful for simple tasks, such as extracting data from text, or more complex tasks, such as understanding intent or emotions from written or spoken words.
4. Design and implementation
The system’s design should consider current web design standards and users’ needs and expectations. Examples include information architecture, navigation, user experience, interaction design, and accessibility.
In addition, you must consider content management systems such as WordPress when designing a platform for AI personalization. Choosing a platform to implement the AI personalization system is also important. The platform should be flexible and scalable.
Newer platforms based on cloud technology provide a range of advantages, from the ability to easily scale the system (and data) to using open standards, which is sharable with other applications. Platforms such as Hadoop, HBase, Apache Kafka, and MongoDB are good choices for AI personalization as they are used commonly in Web-based analytics.
5. Measurement and evaluation
The last step is considering how you can measure the AI personalization system. Most companies use the same metrics for measuring the success of a channel (e.g., the number of new subscribers) as they do for other marketing campaigns.
However, these metrics are not always accurate indicators of success in AI personalization campaigns if some people who interact with the AI personalization system may never actually reach by any marketing efforts or even if they do not like or trust the recommendations. Metrics that are more specific to the AI personalization system may be useful in assessing the impact of the system.
For example, the total number of users reached by the system may need to be a more accurate indicator of success. However, measuring the number of people who received a recommendation for a particular product or service and bought it may be more accurate.